Industry-Leading AI Expertise for Smarter, Faster Growth
Leverage Prolifics’ AI Consulting Services to transform your industry. From healthcare to finance, retail to manufacturing, our Custom AI Solutions enhance efficiency, decision-making, and customer experiences—driving measurable business impact.
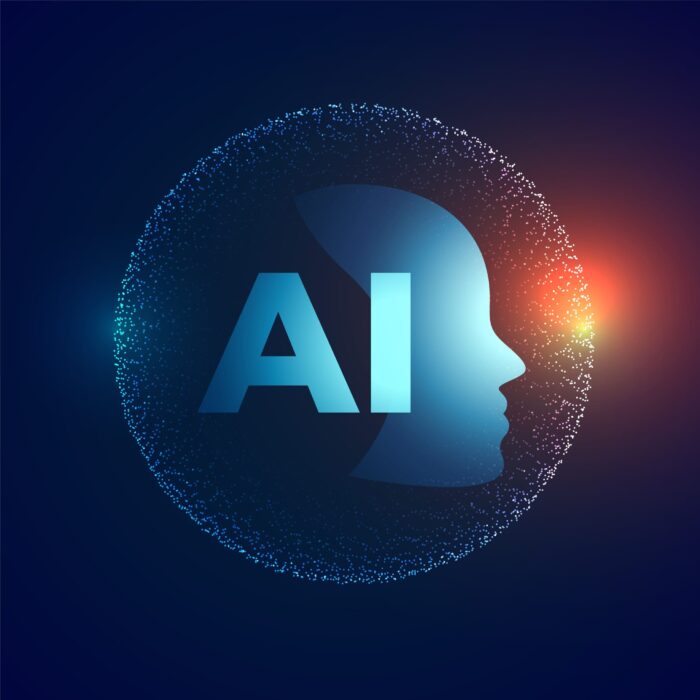
Industry-Focused Solutions Powered by Innovation, Data, and Expertise
Drive business transformation with Prolifics’ industry-specific AI solutions for various industries like Banking, Financial Services, Healthcare, Retail, Manufacturing, and others. Our data-driven, AI-powered automation approach helps you streamline operations, boost performance,and stay ahead in today’s dynamic market. Partner with Prolifics to turn industry challenges into opportunities.
For over 45 years, Prolifics has delivered transformative solutions that create measurable business impact.
With a global team of 2,000+ experts across 10 strategic locations, we provide world-class Custom AI solutions tailored to your needs. Trusted by more than 150 Fortune 1000 companies, we are committed to driving innovation, reliability, and excellence.
Innovation with Impact
Customer-Centric Collaboration
Excellence through Expertise
Generative AI Solutions that Power Smarter Business Transformation
Prolifics leverages Generative AI for Business to revolutionize your business with hyper-personalized customer experiences, optimized resource management, and data-driven market insights. Our Custom AI Solutions seamlessly integrate into your systems, automating complex decisions while ensuring data privacy, security, and ethical AI practices. Partner with Prolifics to unlock Gen AI’s full potential and achieve a lasting AI-Powered Business Growth and competitive edge.
AI-Powered Expertise Transforming Innovation into Impact
Data & AI
Quality Engineering & Assurance
Integration & Platform Engineering
Enterprise Platforms
Intelligent Automation & Applications
Emerging Technology
Driving Measurable Business Success
Our global team of 2000+ experts, spread across 10 strategic locations, ensures world-class solutions are always within reach. Trusted by over 150 Fortune 1000 companies, we deliver reliability and excellence.
Digital Transformation
At Prolifics, we leverage AI-driven Digital Transformation through a structured, outcome-focused consulting approach that ensures strategic alignment, accelerates innovation, and optimizes operational performance.
Intelligent Data Engineering & Generative AI for Enterprise Acceleration
Prolifics’ data engineering and analytics solutions help enterprises become intelligent, connected, and future-ready. We leverage modern data architectures, advanced analytics, and Generative AI for Business to unify data, accelerate innovation, and drive AI-Powered Business Growth. Our scalable, real-time solutions support smarter decisions, seamless integration, and operational agility. From automating insights to enhancing customer experience, Prolifics transforms your data into a growth engine. Unlock the future of your enterprise with AI Consulting Services powered data engineering and analytics.
Prolifics
Where Innovation Meets Expertise
Our Key Partnerships
We partner with the world’s leading technology providers to blend cutting-edge platforms with our AI Consulting Services, engineering, and broader consulting expertise. These strategic alliances enable us to deliver innovative, scalable AI-powered Automation and Custom AI Solutions that drive business transformation, foster growth, and keep our clients ahead in today’s rapidly evolving digital landscape.